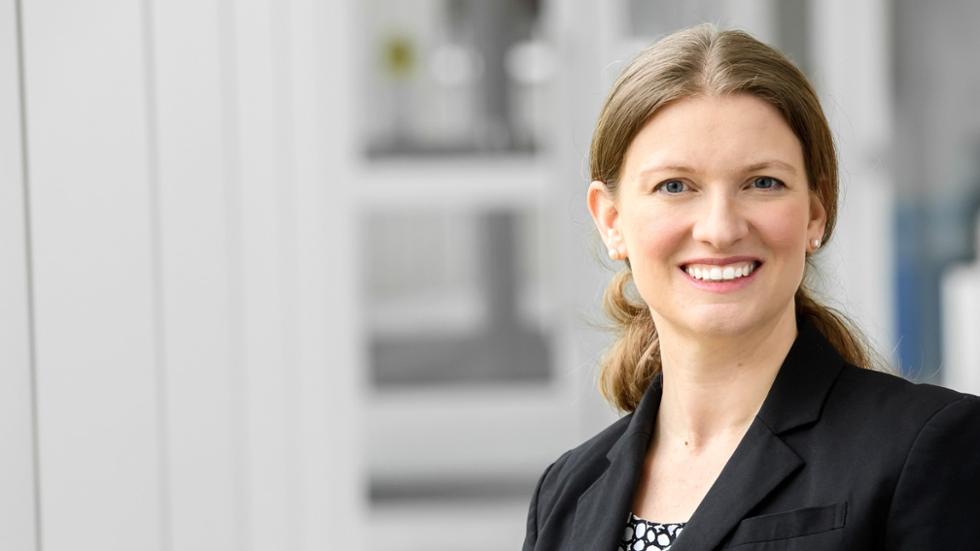
Horowitz said: “We have developed a new way to interpret data from a network of connected resonators to uncover the underlying physical system. It's really exciting because resonant systems show up in a variety of ways: in neural networks, in mechanical systems, in engineered microscale and macroscale devices, But in order to build or use resonator networks, we need to be able to understand the underlying building blocks.”
The abstract goes on to explain: “While these mechanical parameters are typically obtained from response spectra using least-squares fitting, this approach requires a priori knowledge of all parameters and is susceptible to large error due to convergence to local minima. Here we propose and validate an alternative algebraic means to characterize resonator networks with no or minimal a priori knowledge.
Our approach recasts the equations of motion of the network into a linear homogeneous algebraic equation. We employ our approach on noisy simulated data from a single resonator and a coupled resonator pair, and we characterize the accuracy of the recovered parameters using high-dimension factorial simulations. Generally, we find the error is inversely proportional to the signal-to-noise ratio, that measurements at two frequencies are sufficient to recover all parameters, and sampling on the resonant peaks is optimal. Our simple, powerful tool will enable future efforts to ascertain and control resonator networks.”